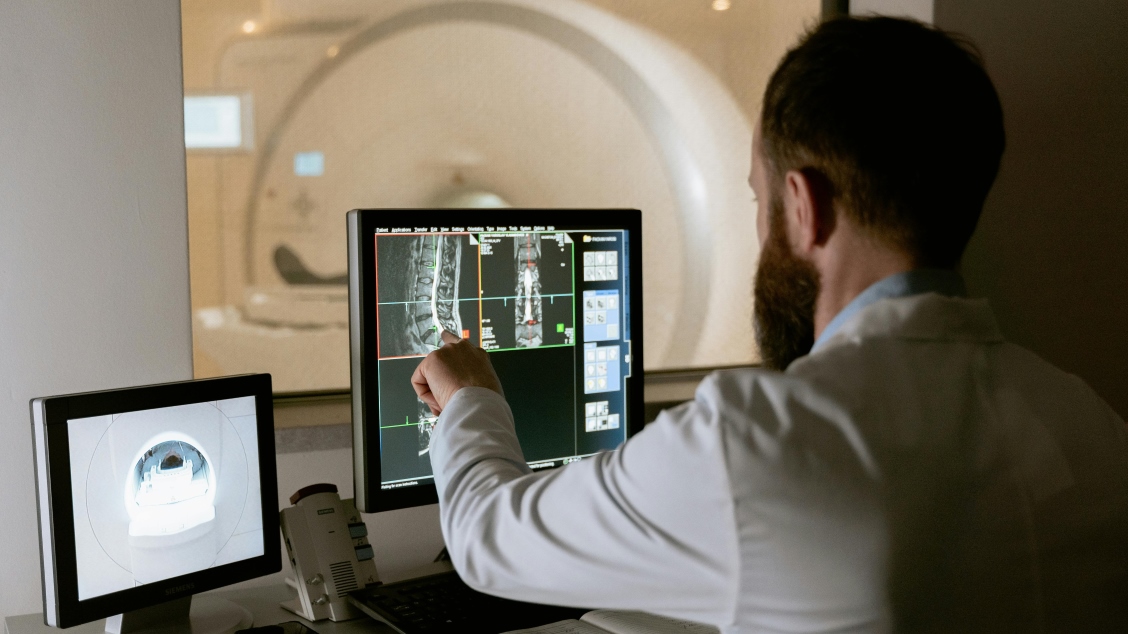
Detecting Brain Tumours Using Deep Learning
Artificial intelligence is reshaping almost every industry. Because healthcare is facing unprecedented challenges, researchers are turning to technological innovations like AI to address them. Here, we’ll be exploring how MDPI scholars are using deep learning to detect brain tumours.
What is deep learning?
Deep learning is a subset of machine learning that uses multiple layers of interconnected nodes to simulate the complex decision-making powers of the human brain.
Models are trained on large amounts of data to identify and classify, recognise patterns and relationships, and make predictions and decisions. Having many layers helps increase the accuracy and improve the models’ capabilities.
What is machine learning?
Machine learning is a rapidly developing field that involves developing algorithms and statistical models that enable computers to analyse patterns in data and make predictions on new, unseen data. Machine learning tools tend to be tailored to perform specific tasks like facial recognition.
If you want to learn more about how researchers are ensuring that machine learning stays precise and safe in science, visit our article Applying AI in Science with Max Tegmark.
Artificial intelligence in healthcare
Globally, healthcare systems are facing several challenges, with populations aging and chronic diseases rising, staff and resource limitations are widespread.
Therefore, researchers are exploring the various ways that AI can support healthcare:
- Administration: automating repetitive tasks, organising data, and creating time for other tasks.
- Cancer patient care: increasing the amount of early and accurate detections.
- AI research assistants: organising data and finding patterns in it, ultimately quickening the research process.
- Artificially intelligent robots: reducing carers’ workloads and helping fight isolation and loneliness.
Challenge of detecting brain tumours
In an editor’s choice article in Algorithms, the authors test a deep learning network’s ability to detect different types of brain tumours in MR images.
The most popular method for diagnosing a brain tumour is by using magnetic resonance imaging (MRI). However, identifying them requires expertise, expensive technology, and time. This is because there are 130 different types of tumours that can affect the brain and central nervous system that change and vary in form, size, and structure.
A timely and correct diagnosis followed by suitable treatment can significantly increase a patient’s chance of survival from a brain tumour.
The authors explain that, with the difficulty of identifying brain tumours, deep learning models are particularly apt for detection. Accordingly, they propose a form of deep learning called a convolutional neural network (CNN) architecture to identify brain tumours using MRI scans.
Why we need to detect brain tumours
A brain tumour is a collection of brain cells that have grown out of control. They are classified into two types:
- Primary brain tumours start in any part of the brain and its related structures. These can be either benign or malignant.
- Secondary brain tumours spread to the brain from somewhere else in the body. These are cancerous and often fatal.
Any tumour expanding in the constrained area of the skull can lead to brain injury and serious issues relating to the different functions of the brain. The authors of the Algorithms article cite that around 250,000 people are affected by brain tumours every year and that this number is rising significantly.
The main treatments are surgery, radiotherapy, and chemotherapy. Quickly and correctly deciding on a treatment plan can save lives.
Detecting brain tumours using deep learning
The authors of the study developed a deep learning algorithm called a CNN architecture.
First, they collected data from an online source and pre-processed the datasets. Then, they applied various machine learning models to train on the images. The dataset was split into three groups: 80% for training; 10% for testing; and 10% for validation.
The CNN was then employed to validate three different types of tumours or no tumour in the images from the dataset. The dataset had a total of 3264 images, which was much larger than in other similar studies.
Promising brain tumour detection using AI
The authors tested the CNN and then compared it with other machine learning models. They tested its accuracy, recall, loss, and area under the curve.
The CNN had an accuracy of 93.3%, which was higher than all the other tested models. They comment:
We may infer that the proposed model is reliable for the early detection of a variety of brain tumours after comparing it to the other models.
For future studies, they describe how the model should be analysed in more detail to understand how it works and to ultimately finetune it. The authors also described how training the model required a lot of computing power, which they did not have, so it took a long time.
Overall, the model demonstrates the high potential of AI and specifically deep learning models in detecting brain tumours and hopefully reducing mortality rates globally.
Read more innovative research
MDPI publishes cutting-edge research relating to artificial intelligence and other technologies that could help us tackle the world’s most pressing issues.
Moreover, MDPI makes all its research immediately available worldwide, giving readers free and unlimited access to the full text of all published articles. It has over 400 journals dedicated to providing the latest findings, many of which publish interdisciplinary research.
So, if you’re interested in submitting to Algorithms, why not look at our list of Special Issues?