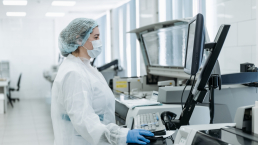
Applying AI in Science with Max Tegmark
Max Tegmark is leading conversations about artificial intelligence. Here, we introduce his work and highlight the exciting articles he’s publishing in MDPI journals on applying AI in science and boosting its effectiveness.
Who is Max Tegmark?
Max Tegmark is a professor of physics at MIT. His research focuses on linking physics and machine learning, which he describes as “using AI for physics and physics for AI”. His 2017 book Life 3.0 discusses AI and its impacts, exploring the variety of societal implications and potential futures AI could produce.
Furthermore, he is the president of the Future of Life Institute, which is dedicated to “steering transformative technology towards benefiting life and away from extreme large-scale risks”. This involves support in policy development, education, research, grant making, and more.
Tegmark’s work on AI won him the Royal Swedish Academy of Engineering Science’s Gold Medal in 2019. The Academy recognised his courageous approach to tackling the existential questions that AI poses and communicating them to the wider public.
Concerns about artificial intelligence
In April 2023, Tegmark featured on the Lex Friedman podcast, which has over 3.5 million subscribers and 550 million views on YouTube (as of 2024), to discuss AI and its dangers.
The podcast centres around an open letter about delaying the development of highly intelligent AI technology whilst safety measures are developed.
Discussing intelligent alien life, Tegmark expressed his belief that we are the only life in the observable universe. Therefore, he argued, we have a lot of responsibility to not mess things up. Life is rare, as is advanced consciousness, so we should care for and protect it.
Moreover, he outlined how much the world revolves around communication, which AI is already beginning to disrupt. He asserted that we’re at an important point in history that could determine the course of the future, and that how we deal with AI is the deciding factor.
Applying AI in science
In short, Tegmark is concerned about AI’s power and capability to disrupt society. In the work he’s publishing with MDPI, we see him addressing these concerns by testing and improving AI’s accuracy when being applied to scientific problems.
Specifically, his work addresses machine learning. Machine learning is a rapidly developing field that involves using computer systems with algorithms and statistical models that can analyse patterns in data. This can enable them to learn and adapt without following explicit instructions.
So, let’s go over his publications with the MDPI journal Entropy and see how they connect to his broader reflections on AI.
Precision machine learning
Firstly, one of Tegmark’s articles explores considerations when fitting machine learning models to data requiring very high precision.
Tegmark and the other authors explain how most machine learning practitioners don’t need to fit data with much precision. This is because traditional applications of machine learning like natural language processing do not prioritise precision. However, machine learning models are now being applied to scientific problems, so the ability to fit models precisely to high-quality data is very valuable.
For example, applying machine learning to healthcare is a case where accuracy and precision can determine lives. Accordingly, the article investigates what considerations arise when attempting to fit machine learning models very precisely to data. The authors call this Precision Machine Learning.
For the investigation, they examine four machine learning approximation methods and study how they scale with increasing parameters and data, identifying errors when they appear.
Comparing models and methods
The article concludes by comparing the four different methods, advising cases in which each method would be best. These include higher-dimensional problems and so on. See the article if you are interested in exploring the cases and methods they advise.
The results highlight both the advantages and disadvantages when using machine learning with scientific data. The article aims to provide useful building blocks for further work in applying Precision Machine Learning. As a result, Tegmark and his collaborators have published vital foundational work that helps guide those seeking to apply machine learning to data.
Accuracy in data compression
In another article, Tegmark and another author address the issue of data compression in binary classification tasks. They explain that a core challenge in science is data distillation. This refers to keeping a manageably small amount of data whilst retaining as much information as possible. Maintaining a smaller amount of data can help reduce storage costs and speed up processing time.
The example he uses is this: what aspects of an image contain the most information about whether it depicts a cat or dog. The aim is to work out the best trade-off so the question of whether it is a cat or a dog can be accurately answered by AI whilst also reducing the largest amount of data.
Additionally, Tegmark and others address data compression in relation to clustering in another article. Clustering is a machine learning method of grouping similar data together. Both articles explore how data compression affects the pattern recognition abilities of artificial intelligence. They offer suggestions in different cases and even modifications to algorithms for those seeking to perform similar tasks.
The importance of publishing Open Access
These articles directly reflect Tegmark’s concerns about the need for accuracy when applying AI to science. Such work helps improve the tools, identify problem areas, and provide a foundation for future work.
In all the mentioned articles, the authors discuss the application of machine learning and their implications for a diverse range of fields. These include neuroscience, linguistics, information theory, and more.
AI applications are not restricted to one field, discipline, journal, or institution. And, by publishing this work as Open Access, it ensures that everyone can access these insights and build on them. This ensures people can use AI effectively and mindfully.
The future of science and artificial intelligence
Nevertheless, concerns about applying AI to science remain widespread. Authors around the world are working on creating safe and effective forms of AI that can help boost science. For example, we covered Four Ways AI Supports Healthcare.
This work is only valuable if it can be seen and built upon. So, if you’re interested in artificial intelligence and its place in the future of science, why not publish Open Access? We have over 400 journals to choose from, including Entropy, AI, and Data. See our full list of journals for more.
Max Tegmark is a leader in discussions about artificial intelligence. In the podcast, he discusses his motivations as stemming from focusing on what he personally finds meaningful and what can help humanity. This is clearly reflected in his work.
He says “the good reason to do science is because you’re really curious and you want to figure out the truth … You know, you stick to what you think is true … You follow your own path”.
It’s becoming clear that with all the brain and consciousness theories out there, the proof will be in the pudding. By this I mean, can any particular theory be used to create a human adult level conscious machine. My bet is on the late Gerald Edelman’s Extended Theory of Neuronal Group Selection. The lead group in robotics based on this theory is the Neurorobotics Lab at UC at Irvine. Dr. Edelman distinguished between primary consciousness, which came first in evolution, and that humans share with other conscious animals, and higher order consciousness, which came to only humans with the acquisition of language. A machine with only primary consciousness will probably have to come first.
What I find special about the TNGS is the Darwin series of automata created at the Neurosciences Institute by Dr. Edelman and his colleagues in the 1990’s and 2000’s. These machines perform in the real world, not in a restricted simulated world, and display convincing physical behavior indicative of higher psychological functions necessary for consciousness, such as perceptual categorization, memory, and learning. They are based on realistic models of the parts of the biological brain that the theory claims subserve these functions. The extended TNGS allows for the emergence of consciousness based only on further evolutionary development of the brain areas responsible for these functions, in a parsimonious way. No other research I’ve encountered is anywhere near as convincing.
I post because on almost every video and article about the brain and consciousness that I encounter, the attitude seems to be that we still know next to nothing about how the brain and consciousness work; that there’s lots of data but no unifying theory. I believe the extended TNGS is that theory. My motivation is to keep that theory in front of the public. And obviously, I consider it the route to a truly conscious machine, primary and higher-order.
My advice to people who want to create a conscious machine is to seriously ground themselves in the extended TNGS and the Darwin automata first, and proceed from there, by applying to Jeff Krichmar’s lab at UC Irvine, possibly.
Thanks for taking the time to read the article and providing your interesting reflections on it. Note, we removed the link from your post.