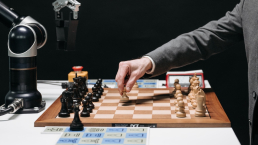
What is Artificial General Intelligence?
Artificial intelligence (AI) has become widely popular since the release of ChatGPT, causing a mix of excitement and concern. Lots of these feelings come from the potential for AI becoming smarter than humans. Some call this artificial general intelligence (AGI), a vague and revolutionary development that has been interpreted in many ways.
The main issue of defining AGI is that it’s based on human intelligence, which is equally difficult to define. Here, we’ll explore the contested meanings of intelligence and how this applies to AI. Furthermore, we’ll explore AGI, the claims that ChatGPT is approaching AGI, what AGI could look like, and what concerns surround it.
Artificial intelligence
AI is a field that applies computer science to robust datasets for problem solving. Common uses include organising and sorting data, generating new data, and recognising patterns, among countless other things.
The term AI provides us with some insight into how it works. ‘Artificial’ simply means something that is made by humans, often as a copy of something natural—in this case, human intelligence.
A good way to think about AI is that it’s trained to mimic human intelligence. It’s not replicating it but trying to replicate patterns and methods that humans perform to achieve certain goals.
Moreover, behind all AI tools are vast human workforces. These workers are known as annotators, performing tasks such as tagging data, labelling images, and performing facial expressions into cameras. Simplifying reality for a machine requires a lot of complexity for humans, as it requires perfect consistency. Evidently, human intelligence forms the basis of artificial intelligence.
ChatGPT’s remarkably natural conversational style is enabled by several rounds of human annotation. This is known as reinforcement learning from human feedback.
What is intelligence?
So, AI tools are enabled by humans—but what is human intelligence?
The term is defined as the ability to learn, understand, and make judgements or have opinions that are based on reason. This encompasses a broad range of processes, instead of a single ability. This is why definitions for intelligence are often highly contested.
For example, two people could be considered intelligent with nothing in common. The first person may be intelligent because of a specific set of skills, which the second person may lack. However, the second person may similarly have a set of skills that makes them intelligent, which the first person lacks. Even though neither set of skills crosses over, and the two have nothing in common, they are both intelligent.
This is where general intelligence comes in.
General intelligence
The concept of general intelligence was developed to deal with this ambiguity around intelligence.
British psychologist Charles Spearman designed tests to measure intelligence. He noticed that people who tended to perform well on one test also performed well on others. And that those who scored badly on one tended to score badly on others.
He concluded that intelligence is a general ability that people can measure and express numerically, like measuring someone’s IQ.
General intelligence can be defined as the existence of a broad mental capacity that influences performance on different cognitive measures.
This is similar to athleticism: a skilled runner may not be a good football player, but because they are athletic and fit, they will probably perform better than someone who is less physically active and is also not a football player in a football game.
Whilst this is a helpful indicator of intelligence, tests like IQ and others are fundamentally flawed, and ignore so many aspects like emotional, spatial, and interpersonal intelligence. Thus, human intelligence remains a mysterious concept, surrounded by lots of debate.
So, what about artificial intelligence?
Today’s AI is highly specialised. A chess program is extremely good at playing chess but cannot write an essay on chemistry. Similarly, ChatGPT is great at producing written text but is very poor at maths. For these tools, their intelligence is limited to one domain.
This is known as narrow AI. A chatbot like ChatGPT produces fluent language because it’s trained on such a large amount of language, but this fluency does not reflect any ability to reason or understand what it outputs. This disconnect holds current AI back from achieving AGI.
What is artificial general intelligence?
Artificial general intelligence has varying definitions, but here are some commons elements:
- Its intelligence is compared to humans, being as good as or better.
- Autonomy and flexibility: the tool can perform on its own and adjust its processes as and when needed.
- The ability to learn from experiences and apply it is very sophisticated.
- It can perform tasks over many domains, adapt to changes in environments, and solve problems it is not trained to.
Testing for AGI
Some argue that common sense intelligence is necessary for AI to achieve general intelligence, but this is challenging. Common sense knowledge consists of facts about the everyday world that humans intuitively or instinctively know. This is because common sense requires fundamental knowledge about how the physical and social world works.
Moreover, there is a view that the Turing test is no longer sufficient, as chatbots have already passed it and that, instead, tests like the coffee test are necessary. This requires an AI “to enter an average American home and figure out how to make coffee: find the coffee machine, find the coffee, add water, find a mug, and brew the coffee by pushing the proper buttons”.
For AGI to pass such tests, it must have embodiment and embeddedness. These terms similarly reflect a physical and sociocultural integration into the world and require a high degree of contextual awareness. They involve visual and spatial awareness, knowing what objects are and what they do, how to use them, and how they interact, amongst many other things.
These tests highlight how fundamental and intuitive intelligence in humans is very hard to replicate in AI, harder than making an AI an expert in chess. However, already, we seem to be moving away from the technical questions to a more philosophical and value-driven conversation.
Can machines be intelligent?
AGI requires engagement between a highly technical scientific field and some of the most profound and longstanding philosophical debates about human intelligence and consciousness. It requires a re-evaluation of notions debated by ancient Greek philosophers and reflection on ourselves as much as it does about the capabilities of AI.
But what if we’re asking the wrong question? Defining intelligence has stumped thinkers for millennia now. This is what an author in the MDPI journal Computer Sciences and Maths Forum explores in a Proceeding Paper. They use three philosophical approaches to challenge the idea of AI even being intelligent.
Arguing against artificial intelligence
The authors believe large language models like ChatGPT are setting the agenda for expectations around AGI: highly functional machines that can interact with us using sophisticated language.
However, they argue, artificial intelligence is not intelligent. AI tools are based on algorithms. Algorithms represent a strict determinacy; by this, they mean they are fixed and exact, with highly complicated variations and vast combinations of if x, perform y. Because they are so rooted in human-determined algorithms, the authors argue they cannot bring about meaningful information by themselves and are therefore not intelligent.
For the author, the debate stems from us incorrectly anthropomorphising AI, namely, giving it human features. But AI requires a redefinition of common terms we apply to humans.
For example, autonomy in humans refers to freedom in action, but also in morality, emotions, and thus accountability. For machines, the authors explain, autonomy refers to acting and operating independently through a consideration of order generated by laws and rules, an order that is implemented by the human creators.
Consequently, we get back to this idea of machines mimicking human intelligence, rather than replicating it.
Concerns about artificial general intelligence
Regardless of whether these arguments are sound, or you fully align with them, they reflect the ambiguity around the concept of intelligence in both humans and AI. Moreover, they require us to sharpen our definitions and face the inconclusiveness of our understanding of intelligence—for example, how different are our neurological networks to an AI’s algorithms?
Keeping this ambiguity in mind, concerns about AGI can move from the philosophical realm to one that’s more practical. What are the main practical concerns about AGI?
Self-programming
The defining event that would likely trigger AGI regardless of definition is self-programming or -improving AI. Essentially, this involves AI no longer requiring the human element of the reinforcement learning process and improving its algorithms itself.
Lots of fear around AI is rooted in this scenario, famously described in the paperclip paradox. These apocalyptic concerns often ignore the wide range of work being done on developing guardrail technologies for AI tools and implementing international AI regulation.
Biases and errors
We’ve previously discussed biases and hallucinations in generative AI and how they can cause the spread of misinformation. A recent example was Google Bard sharing incorrect information about the James Webb Space Telescope taking the first ever picture of an exoplanet.
When implemented into other tools and systems, the results can be graver. Here are two examples.
Examples of AI errors with consequences
First, in 2020, it was revealed that UK passport photo checkers show bias against dark-skinned women. These women were more than twice as likely to be told their photos fail passport rules when they submit them online than lighter-skinned men.
This example shows how, despite training and checks, longstanding racial biases can get through. Discrimination can be built into how we categorise and measure data and be practised by machines.
Second, errors in artificial intelligence can lead to the loss of life. In 2018, a self-driving Uber car killed a woman crossing the road with her bicycle. The AI had been trained to stop when it sees someone crossing a road and to recognise that someone on a bike is indeed a person. However, the AI was not trained to recognise someone walking with their bike whilst crossing the road. This is known as an edge case—an encounter that is not well represented in the data.
These two examples highlight the potential for AI to incorporate biases or errors because of issues in data. With issues potentially stemming from long-standing discrimination or oversights, there are already real dangers from implementing tools before they are ready.
Reflecting on artificial general intelligence
Conversations about artificial general intelligence often turn philosophical. This is because the concept of intelligence is elusive and hard to define for humans. Since AI is mimicking, not replicating, human intelligence, it’s easy to get lost in abstract reflections rather than looking at AI and what it can actually do.
For now, tools like ChatGPT are not AGI, despite how impressive they seem. They are narrow AI because they perform specific determined tasks and cannot step outside their domain, as wide as it is, nor understand it.
For tools to achieve what could arguably be AGI, they need self-programming and/or the development of contextual awareness, measured using tests like the coffee test. Focusing on practical and measurable elements of AGI would help make it easier to recognise.
Furthermore, issues like biases and errors are already prevalent in AI and, as shown, can have very real consequences when implemented. So, it is important not to let current problems get shadowed by speculation about the future or debate over categorising intelligence.
AI research
Addressing biases and working to make AI empower our productivity and engagement could be what’s necessary to challenge those apocalyptic scenarios like in the paperclip paradox.
At MDPI, we are very interested in exploring AI’s capabilities and ensuring it works in our best interest. MDPI makes all its research immediately available worldwide, giving readers free and unlimited access to the full text of all published articles. Open Access is vital for ensuring we develop AI collaboratively and safely.
Moreover, we’re very interested in exploring AI here on the Blog. In a recent article, Applying AI in Science with Max Tegmark, we explore how a leading voice in AI ensures the safe implementation of AI in scientific research by exploring his research published by MDPI.