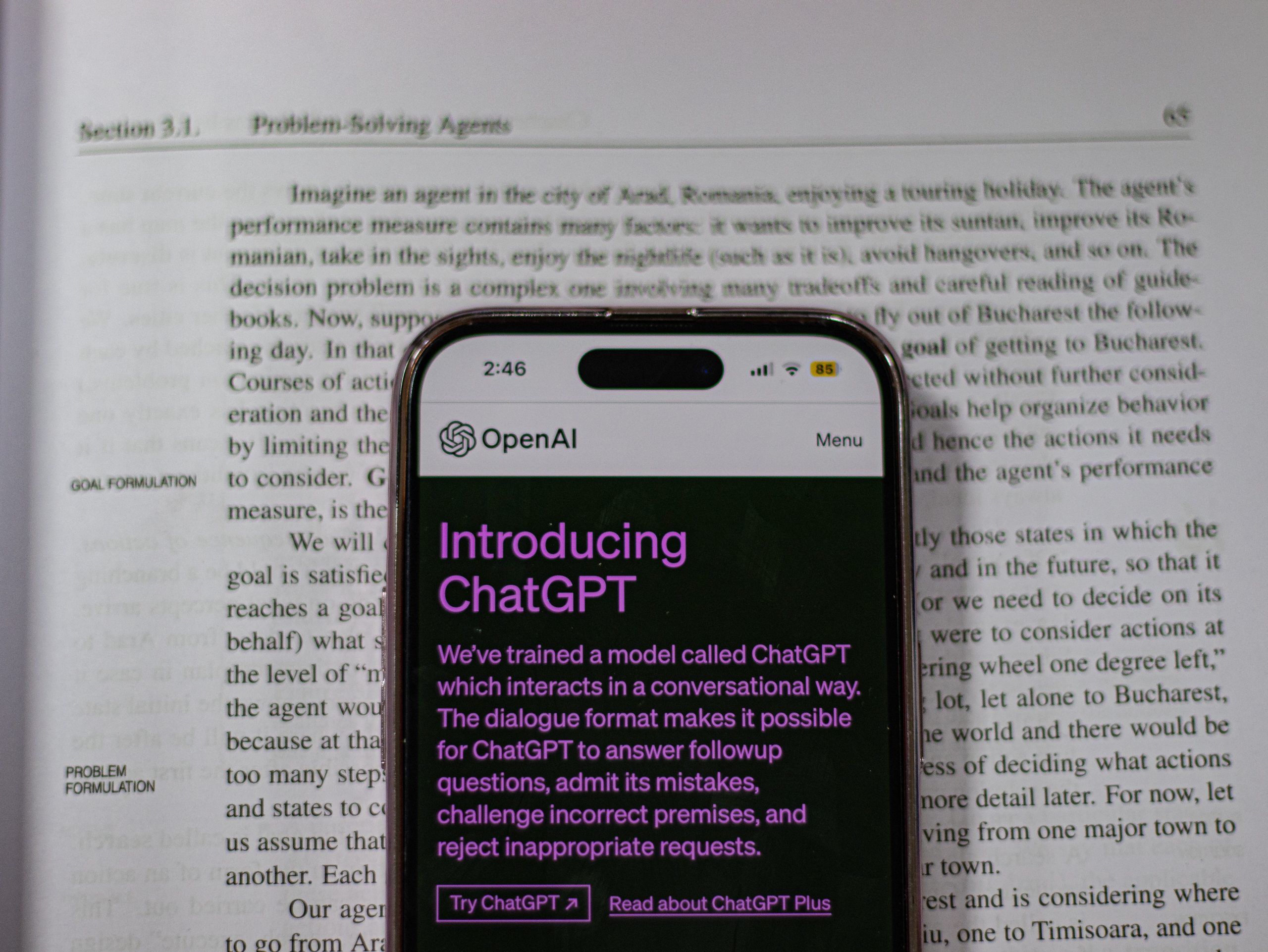
Artificial Intelligence: Ethical Considerations In Academia
The recent developments in the field of Artificial Intelligence have generated a lot of excitement and uncertainty. Generative AI and, in particular, large language model (LLM) chatbots that can be used to easily create original content are widely debated. Most acknowledge that they can be good or bad depending on how they’re used.
One area where there is widespread concern is academia and education. Whilst programmes like ChatGPT can be a helpful tool for research, they also present many issues regarding ethics in education and academic integrity.
Here, we take a closer look at LLMs and the ethical considerations of AI in academia.
Overview of mechanism
LLM AI models—such as ChatGPT—use natural language processing to analyse and learn patterns in human-created texts. As they are trained on large datasets, which cover an array of topics, they can generate responses that appear comprehensive and well-researched.
They generally take the form of chatbots, which are able to rapidly generate responses to a wide variety of prompts inputted by the user. The results are largely indistinguishable from human responses. Such models are capable of producing texts that appear to consider issues from multiple angles and perspectives.
They can be used to answer specific questions, generate ideas, summarise texts, translate languages, generate full texts, and more.
Accuracy of LLM Artificial Intelligence Models
Though their conclusions may look well-reasoned, there is concern about and wild fluctuation in LLMs’ accuracy.
One study found that ChatGPT’s accuracy varied, from 4.8% to 26%, depending on the type of prompt. This is partially because it struggles with certain components of natural language, such as negatives or words such as ‘only’ and ‘alone’. Also, for topics for which there is a lot of competing training data, LLMs become ‘confused’ and have been shown to agree with falsehoods.
This is because large language models lack the capacity for systematic reasoning. They don’t understand the datasets they are trained on, limited to purely identifying patterns in the communications of humans. The nuances of language, therefore, are lost on programmes like ChatGPT, and they are unable to think critically about the information they are presented with.
Further, ChatGPT specifically is trained on datasets created up to January 2022. So, any information from that point on will not be factored into its conclusions. This is particularly an issue in the sciences, where keeping up to date with research is crucial for drawing scientifically accurate and valid conclusions.
Also, LLMs have no expertise in a specific field. Whilst they can give broad summations, ultimately, they are not specialised researchers and cannot be relied upon to present accurate conclusions. They also suffer from “hallucinations”, generating text that is coherent and looks plausible, but which has been completely made up.
The black box effect
The black box effect is one of the biggest concerns in the field of AI. A black box problem is one where the inputs and outputs are known, but the method by which the outputs are produced is unknown.
With regard to AI, we are able to set parameters for it to consider and review the conclusions. However, we have no idea about the methodology by which it draws these conclusions. Due to this lack of transparency, we are unable to interpret AI algorithms. Artificial Intelligence is increasingly being used in decision-making procedures, but we are completely blind to the process by which it arrives at its conclusion.
Without a window into AI models’ methodology, how can we be certain that it is unbiased and ethical? There is a lot of inherent bias in the datasets AI is trained on; being written by humans, many texts will be opinionated, unfair, or misinformed. How, then, can we expect its conclusions to be free from bias?
Furthermore, who is responsible for AI-generated mis- or disinformation? If a person does not fact-check the information provided by, say, ChatGPT, are they then responsible for spreading false information or is the AI programme itself to blame?
Ethical considerations of artificial intelligence in academia
There are many ways in which LLMs can facilitate research processes and learning: they can be used to summarise complex texts, generate prompts, complete time-consuming and repetitive administrative tasks, analyse data, etc.
They can also be used to generate complete texts, such as essays and whole theses, which is cause for serious concern. Many think this could have a huge impact on academic integrity, as well as affect the competence and skills of students and researchers.
Below, several ethical perspectives are considered regarding the use of generative AI in academia.
Bias, fairness, and transparency
As previously discussed, AI has limited critical thinking faculties. It is incapable of reflecting on the information it consumes. Nor can it review its conclusions and decision-making processes the way a human can.
With humans being unable to review these processes in place of AI, it is impossible to determine if conclusions have been drawn fairly or whether biased, outdated, or problematic material informed the final decision.
It is imperative that fairness is protected and that AI models do not feed into societal stereotypes. Bias needs to be addressed in many of these systems, which is currently difficult due to the black box effect.
Therefore, researchers using AI to produce academic material, then, could unintentionally be further perpetuating biased research or opinions. Or, they could be disseminating information that is completely fabricated.
There are also many issues with automated decision-making procedures being susceptible to bias. Automation could lead to unfair treatment in many areas of society. For example, bank loans, criminal justice, hiring processes, university admissions, and so on.
Plagiarism
Plagiarism, presenting others’ ideas as your own without proper attribution, is a serious form of academic misconduct resulting in disciplinary action by universities. In the past, it was assumed that one can only plagiarise other humans. With the rise of Artificial Intelligence, however, this is no longer the case.
Currently, it is impossible to reliably identify work crafted by generative AI models. This is because often the work created is completely original. And, as they mimic human language so well, there are few markers that suggest the results were created by AI.
Many suggest that, in their current iteration, programmes such as ChatGPT will produce earlier, less detailed academic essays successfully. But, students will find less success using it as the work becomes more specialised.
Educators are encouraged to look out for any glaring changes in language or style between individual students’ papers. Also, one marker of AI-generated content to be alert to is excessive use of facts with “little critique” by the author.
Misinformation
LLMs are programmed to provide information by searching databases and making guesses based on the most suitable results and probability. Unlike search engines, they do not present you with the list of most relevant sources so you can review them yourself; they present their conclusions as fact.
In academia, where sources must be diligently referenced and justified, this is a concern. Taking an LLM’s conclusions at face value, with no reference to the source material, can promote the dissemination of falsehoods.
There are also many reported instances of ChatGPT presenting its own ideas as research-backed facts. It may not just be giving credence to misinformation from humans, but could be actively contributing its own fabricated research in any given field.
Influence of artificial intelligence on researchers
With the automation of research tasks, many are concerned about the erosion of research skills. Whilst appealing in terms of saving time and energy, future researchers may become reliant on LLMs. Thus, there could be a decline in their competence and quality of research.
Academic writing and language editing
A highly important area for academic competence is written communication. Students utilising LLMs such as ChatGPT to write parts of or even whole essays is a serious problem not just in terms of plagiarism, but also in terms of honing abilities that are fundamental to conducting fair and valid research.
LLMs are also being used to edit various academic works—from first-year essays to published articles. As previously mentioned, they are unable to think deeply about information. Whilst they can make some sophisticated changes to language, grammar, and style for some forms of content, they don’t have the same robust academic editing experience as professional editors. Nor do they have the same proficiency as native-level speakers.
Authorship and AI at MDPI
MDPI is committed to research integrity and the ethical use of AI and AI-assisted technology in manuscript preparation. Large language models (LLMs) such as ChatGPT do not meet authorship criteria. Thus, they cannot be listed as authors on manuscripts.
Authors are required to be transparent about the use of these tools. Details of how the AI tool was used must be disclosed in addition to providing the AI tool’s product details.
Authors are responsible for ensuring that their manuscripts comply with MDPI’s publication ethics policies. Read more about Authorship and AI at MDPI here.
The future of AI in academia and education
Whilst there is a lot of opportunity for AI in various learning and research processes, we must proceed with caution.
Regulations and tools must be developed to ensure that AI is used responsibly and in a way that guarantees freedom from bias. At the moment, LLMs are only as good as the datasets they are trained on. Therefore, there is no guarantee that their conclusions are scientifically valid or accurate.
To learn more about Artificial Intelligence, take a look at the research of AI and previous blog articles.
Thank you for the comment about AI’s importance we appreciate you.
Hi,
Thanks for taking the time to read our article and leaving this comment.
All the best.
This article is very interesting and has opened my eyes in various ways wrt AI.
Hi,
Thanks for taking the time to read our article and leaving this comment.
All the best.
La claridad con la que has abordado los conceptos técnicos y teóricos hace que este artículo sea una excelente referencia para estudiantes y profesionales.
Hello,
Thank you for your comment and kind words.
All the best.
Thank you for the insightful piece on AI in academia! It’s crucial to embrace this technology and prepare for an AI-powered future. Your discussion underscores the importance of leveraging AI to enhance educational practices while being mindful of ethical considerations.
Hi,
Thanks for your comment and reflection on AI’s importance! We removed the link from your comment, I hope this is not an issue.
Thanks.
Hello Jack,
Thank you for your kind words and yes definitely there is not an issue with removing the link.