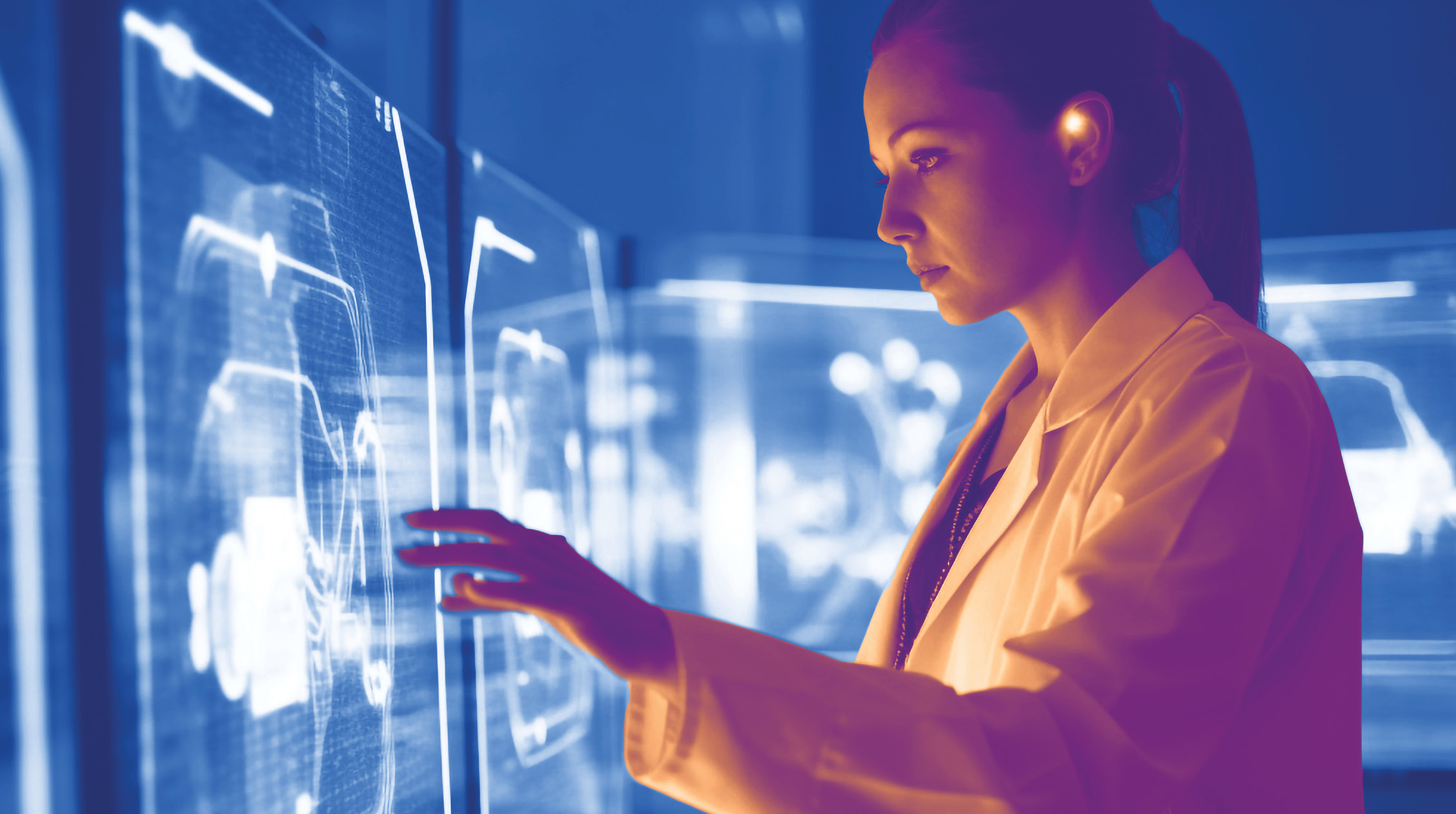
Applying Digital Twins in Healthcare
Digital twin technology has the potential to revolutionise personalised medicine and improve the efficiency of public health organisations. Here, we explore applications of digital twins in healthcare and the resulting ethical concerns and technical challenges.
What is digital twin technology?
A digital twin (DT) is a virtual copy of a physical object or process. This could be a patient’s organ or a hospital environment. The DT dynamically updates using data from sensors and sources, like electronic health records, heart monitors, and public registries, so it reflects the physical version as it undergoes changes.
A DT constitutes three parts:
- Physical object.
- Virtual object: representation of the physical object.
- Digital thread: the flow of data between the two.
In manufacturing, digital twins are useful for simulating, monitoring, and controlling the state and performance of machines. They are a dynamic tool to analyse and optimise an object or process. Moreover, they can help to lower cost and risk as more testing can be carried out digitally, without using physical resources.
Click here to learn more about Integrating Digital Twins in Manufacturing.
Digital twins in healthcare
Let’s explore the potential of using digital twins in healthcare to aid in personalisation and efficiency.
Personalised medicine
Personalised medicine, also known as precision medicine, avoids a one-size-fits-all approach and looks at a patient’s genes, environment, and lifestyle when developing treatment and disease prevention strategies.
Essentially, personalised medicine recognises that not all patients respond in the same way to the same treatment, with genetics being one example of something that can drastically influence responses.
Digital twins could revolutionise personalised medicine by creating virtual copies of patients. These could range from a single organ to the entire body. These would be created and updated using data sources like sensors, routine blood tests, and self-inputted data, among others, to highly personalise health plans, particularly for people with chronic diseases.
As a result, a digital twin of a patient could be genuinely personalised, using vast amounts of public and personal data about their genes, proteins, cells, and whole-body systems. Healthcare professionals could use a patient’s DT with tools like machine learning, a form of artificial intelligence, to predict the best possible treatment. This is especially valuable for cases in which you need to reduce risk, such as for people with cancer or who are pregnant.
Let’s look at a specific example of this in practice.
Managing elderly type 2 diabetes
An article in the Journal of Clinical Medicine created a human digital twin for personalised elderly type 2 diabetes management.
The authors explain that managing elderly type 2 diabetes (E-T2D) is challenging due to it being paired with other geriatric conditions and lifestyle factors, such as reduced activity levels, multiple drug intake, and variability in nutrient absorption. This makes their blood glucose levels highly unpredictable. Therefore, personalised medicine and treatment are essential.
The authors created DTs of 15 patients with E-T2D to deliver precision insulin and gain deeper insights.
They found that personalising diabetes treatment using DTs enhanced the time-in-range (the amount of time blood sugar is within the target range), reduced the number of hyper and hypo events (when blood sugar is too high or too low, respectively), and reduced the amount of insulin infusion needed by 14‒29%.
This small study showcases that DTs are highly promising for managing E-T2D and how they could be applied to managing chronic diseases.
Public health
Digital twins can also be used on a broader scale by replicating healthcare centres and their processes to identify and solve problems. Optimising the organisation of health institutions could save lives and respond to the various issues hospitals are facing:
- Growing patient demand.
- Increasing clinical complexity.
- Aging infrastructure.
- Lack of space.
- Increasing waiting times.
Different possible solutions can be tested in virtual environments before being implemented in real settings, such as bed planning, staff schedules, and even sharing resources between hospitals. This could reduce risk and costs when making changes in fast-moving and high-stress environments.
Efficiently mapping out the resources of different hospitals in an area is vital for ensuring patients are sent to the right place at the right time. For hospitals struggling with overload and outdated processes, improving efficiency in ways like this could vitally help save lives and lower costs. And it could improve patient service and safety.
Furthermore, DTs can be used to simulate crises to boost preparation and management. Machine learning can predict the outcomes of crises, like a sudden, high influx of patients or specific outbreak of a disease, to create plans for distributing resources and patients and find potential weaknesses in the infrastructure.
In short, implementing digital twins in healthcare settings can help respond to challenges without having to take any immediate risks and also aid in the preparation and management of crises.
Supporting health in cities
Furthermore, digital twins can also be used in conjunction with city management.
In Boston, digital twins are used for proposed buildings to visualise how much sunlight they would block. This helps prevent large shadows increasing vitamin D deficiency risk in locals, particularly in winter. Vitamin D deficiency symptoms include frequent illnesses or infections, fatigue or tiredness, bone and back pain, and depression.
Furthermore, DTs can be used for tracking and managing disease outbreaks to better prepare and respond to them. Using data on disease cases, a dynamic map with information on high disease transmission risk areas can be created to provide real-time alerts to locals. Simulations of different responses and events can be used to create plans for different scenarios and to highlight weaknesses in infrastructure.
To summarise, DTs can be used in city management to create spaces that do not negatively impact citizens’ health and also to prepare for and respond to disease outbreaks.
But what are some of the concerns about using digital twins in healthcare?
Barriers to using digital twin
A review in the Journal of Personalized Medicine reflects on the barriers hindering the larger and faster adoption of digital twins in healthcare.
Ethical concerns
There are range of ethical concerns about embracing DT technology, particularly because it intersects with concerns surrounding other emerging technologies like AI and big data. Here are some examples that the authors cite:
- Security and privacy: patient data includes highly personal biological, physical, and lifestyle information. Therefore, confidentiality is highly important. However, there are concerns about who has access to this information, such as insurance companies, and cybersecurity risks.
- Data biases: many datasets incorporate racial, gender, or other demographic sources of bias. DTs need to be designed carefully so they do not intensify existing biases.
- Accessibility: embracing DT technology will initially be highly expensive and require the development technological of infrastructure. This could widen already existing socio-economic gaps between and within countries.
- ‘Good’ gene pool: human body DTs intersect with broader concerns about eugenics, which refers to discrimination based upon inaccurate theories related to genetics.
Technical limitations
Furthermore, DT technology requires highly sophisticated technical infrastructure and competence, which poses a few challenges for its adoption:
- Data collection and management: electronic health records need to be highly sophisticated and detailed. Establishing this can be expensive, time consuming, and require expertise which may be lacking in healthcare settings.
- Process and interface design: interdisciplinary knowledge is needed to construct interfaces and tools that are applicable across different specialist fields of knowledge, such as biomedicine, genetics, mathematics, and computer science.
- Training and costs: healthcare settings dealing with a lot of the aforementioned difficulties, like underfunding or long waiting lists, may struggle to introduce new, complex technology that requires training and integration.
Digital twins in healthcare and more
In short, digital twin technology poses ethical questions regarding patient privacy and the potential for discrimination and technical limitations as it requires highly sophisticated and complex technological infrastructure.
However, as we explored above, digital twins in healthcare presents opportunities for revolutionising personalised medicine and improving efficiency and crisis preparation in public health settings.
Overall, digital twin technology is an emerging field with large amounts of investment and research. Hence, we are likely to see significant advances in DT technology and movement towards responding to the ethical concerns we’ve outlined.
Timelines will vary as to when and where organisations adopt DT technology. Currently, much like drug trials and tests, examples of DT are in the proposal, testing, or research stages, and will gradually be implemented in specific cases before a widespread adoption after sufficient testing.
At MDPI, we are interested in exploring DT’s capabilities and showcasing exciting uses of it. If you want to learn more about DT, why not begin with our article What is Digital Twin Technology?