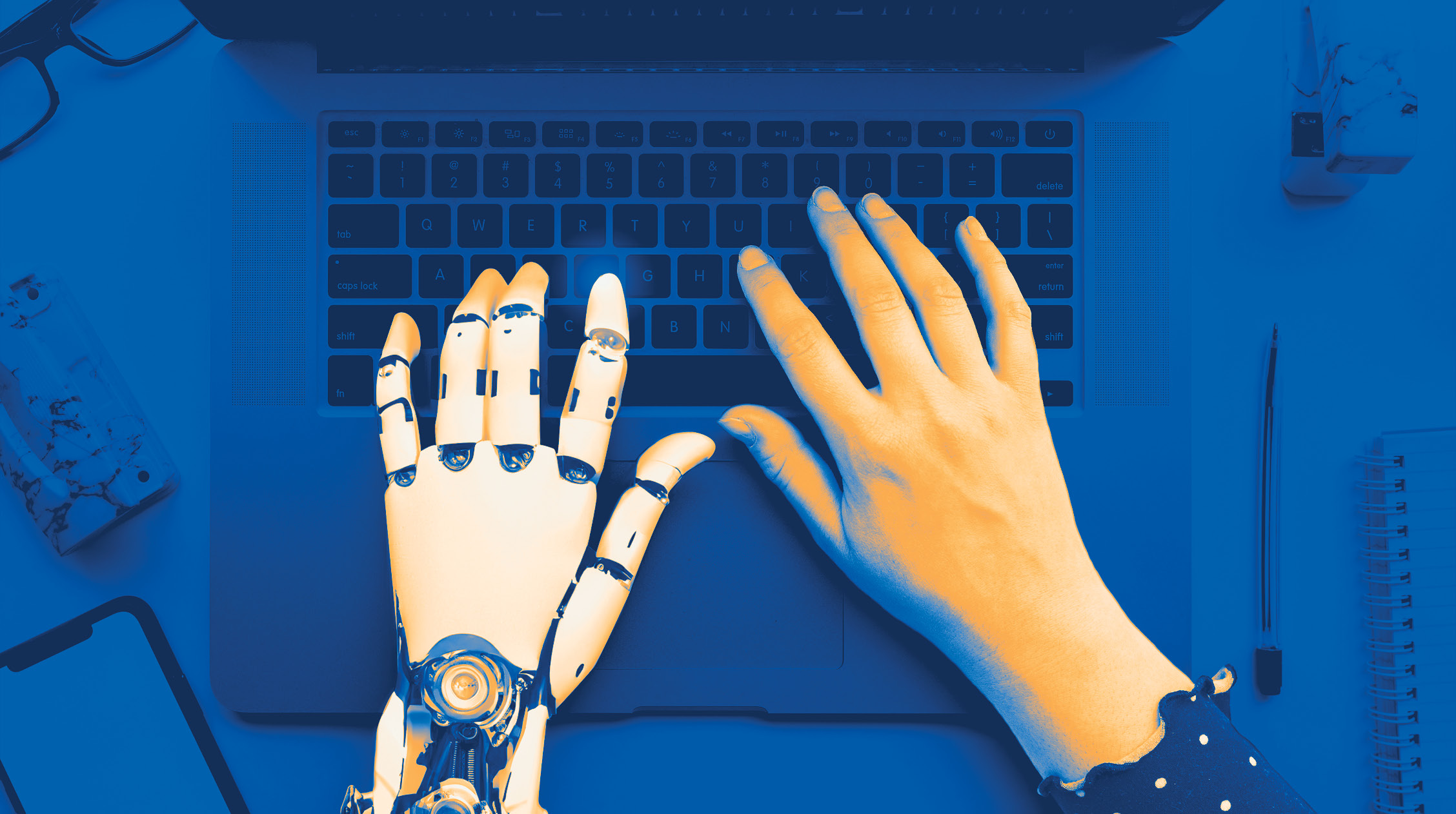
Artificial Intelligence in Scientific Publishing
In 2023, artificial intelligence swept the world, raising interest and concerns over its revolutionary potential in most sectors. In 2024, we’ll see further integration of artificial intelligence in scientific publishing, as the industry begins to adapt to the new technologies.
Here, we’ll go over AI’s effects on scientific publishing and the challenges this industry is facing.
What is artificial intelligence?
Put simply, AI is a field that applies computer science to robust datasets for problem solving. This can come in the form of organising and sorting data, generating new data, and recognising patterns, among many other things.
The key factor of AI is that it can learn and adapt from its experiences. We see this in self-driving cars, facial recognition software, and chatbots.
Generative AI
In 2023, generative AI tools, most famously ChatGPT, boomed in popularity. These are machine-learning models that can generate new content.
GPT stands for ‘Generative Pre-trained Transformer’. The ‘Pre-trained’ refers to how the model is trained on a large corpus of text data to predict the next word in a passage. This is so it can produce human-like text. It works similarly with other outputs, including images, music, animation, or code. It ultimately aims to produce statistically probable outputs.
GPT is an example of a Large Language Model (LLM), which are broadly designed to understand and generate human-like text. LLMs are trained on human text to generate textual content. All LLMs are forms of generative AI.
Why is AI-generated text so difficult to detect?
Tools like ChatGPT are one of the main discussion points about artificial intelligence in scientific publishing. We asked Jean-Baptiste de la Broise, a Data Scientist for MDPI’s AI Team, why AI-generated text is so difficult to detect.
He explained that, to make a human-sounding Large Language Model, you need to introduce some randomness in the replies, like how a human will rarely reply in the exact same way to a complex question. He explained:
This implies that, for any input (prompt), the LLM can generate many different outputs (among which some are very close to how a human could have replied).
He also outlined some additional factors:
- The options for inputs and outputs of LLMs are infinite.
- The detection models’ accuracy depends on the length of the text, meaning the shorter the sample, the more difficult it is to detect whether it is AI-generated.
- A slight modification of an LLM output may deceive the detector, as detectors are adjusted to the current versions of LLMs.
- There are many more resources being invested in the development of LLMs than in the detection of AI-generated text.
- The diversity of LLMs means it is difficult to detect all LLM outputs (even if there are commonalities between the different LLMs, as they often train on similar data, use the same underlying architecture, and optimise towards the same objective).
How publishers are responding to artificial intelligence in scientific publishing
The detection of AI-generated content remains a pressing issue in scientific publishing. Publishers permit the use of generative AI at varying degrees, provided it is noted in the Acknowledgements or Materials and Methods sections. However, regulations vary between publishers.
For review reports, the use of AI tools is generally not permitted. This is because inputting the review report or parts of the written manuscript into the AI tool would be a breach of the confidentiality clause between the reviewer and publisher as the text is technically being shared with a third party (i.e., the AI tool, which may store inputs).
MDPI’s views on the use of AI
Regarding AI tools in manuscript preparation, MDPI follows the Committee on Publications Ethics (COPE) position statement. Tools such as ChatGPT and other large language models do not meet authorship criteria, primarily because they cannot be held accountable for what they write, and thus cannot be listed as authors on manuscripts.
In situations where AI or AI-assisted tools have been used, this must be declared with sufficient details via the cover letter, with transparency about their use in the Materials and Methods section and product details provided in the Acknowledgements section.
But why do authors feel inclined to use AI tools?
Advantages of AI for authors
There are various time-saving and efficiency-boosting benefits of using AI for authors.
Data management and automating tasks
The amount of data being generated and published far exceeds what anyone can read or effectively manage. AI can be used to automate certain data-related aspects of the publishing process:
- Keyword searching for suggesting relevant journals and search engine optimisation.
- Analysis of datasets to generate insights and identify new research areas.
- Processing data for content aggregation in databases.
- Providing advanced search options in databases.
- Checking for and removing duplicate articles in databases.
- Correctly labelling and organising articles.
Also, data is fuel for AI, improving its accuracy and ability, so the more data it processes, the more it should improve.
Translation
Now, many online translators incorporate AI. Google Translate has AI-powered features that enable it to learn and adapt the more it’s used. AI models like ChatGPT can make translating more interactive by using Natural Language Processing (NLP).
NLP applies computational techniques to analyse human language and produce human-like responses. Having trained on so much natural text means that tools like GPTs can provide contextual awareness to translations.
Furthermore, their conversational format means that researchers can ask for clarity, synonyms, or alternative translations to words and phrases. Or they can ask the AI tool to revise the text and adapt the writing into an academic tone.
Therefore, AI tools can make translation more like a conversation, helping to breach the gap between native and non-native English speakers when researching and even writing work.
Paper summarisation
Generative AI can be used to produce summaries of papers. Such tools are useful during the research and writing stages.
During research, AI tools can summarise papers into their main points, which is especially helpful for long articles or ones that are not entirely relevant. The AI can search through a paper and gather all the relevant and key points for a reader, saving time and energy when researching.
Also, paper summarisation can be applied to an author’s own papers. This helps in summarising the article’s key points for writing conclusions, abstracts, and even making presentations about the work.
Image generation
AI can be used to generate images quickly and effectively.
This can include generating representational graphics through written prompts to further boost clarity and exemplify points.
Furthermore, it can be used with data to produce graphs and tables. Both examples could save large amounts of time and even help to improve the clarity of the writing.
Disadvantages of AI in academic publishing
AI poses some concerns for the academic publishing industry.
Difficulty in detecting generative AI
As mentioned, GPTs work by producing human-like text by predicting the next word in a passage. Therefore, GPTs produce text that sounds but may not necessarily be true. This is like how an AI image generator will create an image that looks real, not one that is real.
Not only are GPTs prone to political biases but they sometimes produce hallucinations. This is where the bot confidently makes a plausible-sounding but factually incorrect claim. This can make detecting AI-generated writing difficult, especially when covering topics that writers are not specialists in.
AI models for detecting generative AI
Currently, there is a race between developing generative AI and models that can detect AI writing.
Various guides and tools are appearing online for detecting AI, but because the field of AI is so dynamic, these tools can quickly be outsmarted or even become obsolete. Moreover, there is always room for error using detector tools.
This can lead to fake scientific papers getting published as they can appear very well written and sophisticated in their arguments. This highlights the importance of peer review.
Paper mills
AI-generated texts amplify a problem already prevalent in scholarly publishing: paper mills.
Using AI to generate articles can rapidly speed up the production of paper mills and make their output seem more believable.
Artificial intelligence in scientific publishing
In 2024, AI is only going to grow in capabilities and application. And, in a few years, AI will become the norm, like how the Internet or Google are now. Therefore, after this phase of readjustment, it’s likely that AI will be assimilated and accepted into our use of technology.
Whilst this presents us with a new set of challenges within scientific publishing, it also provides us with many opportunities to improve the editorial process that will likely evolve scholarly publishing.
Frank Sauerburger, AI Technical Leader, summarises the significance of this moment:
Large Language Models and related technologies enable a plethora of new applications and products. AI, and especially Natural Language Processing, has advanced so rapidly in the last few years, that it feels like we are witnessing the beginning of a new epoch.
MDPI and AI
MDPI’s AI team are developing and deploying a range of tools to help address concerns about detecting AI-generated content and dealing with the large volume of articles being produced. They seek to generally help and assist us during the editorial process to improve the quality of our journals.
If you’d like to learn more about MDPI’s AI tools, see our article AI Tools for Researchers in Scientific Publishing.
This article was written in collaboration with Enric Sayas, Scientific Officer and AI Team Business Analyst.