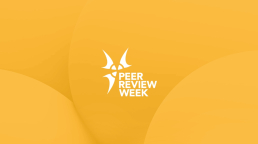
The Growth of Data and Future of Data Peer Review
Peer Review Week 2023 is dedicated to the theme “Peer Review and the Future of Publishing”. Scholarly publishing is transforming at an accelerating pace, because of the growth of Open Access, AI tools like ChatGPT, and diverse research outputs. These three factors are contributing to the growth of data as non-article research output. What does data peer review look like?
Data is an opportunity and challenge to peer review. It’s what supports and underlies most research. And it is fuel for AI, providing material for AI to analyse and make predictions from. But data comes in many diverse forms and requires a multidisciplinary approach to fully address.
So, here we delve into how and why there’s been this growth in non-article output, specifically data, how it’s changing science, what it means for peer review, what it looks like in practice, and the challenges it presents too.
Growth of non-article research output
In 2000, the digital object identifier (DOI) was introduced to help identify and standardise unique digital objects. A DOI was obtained by registering with an agency that ensures the object is entirely unique. This helps with avoiding duplicates and providing easier access to the ever-growing literature in content aggregators.
In the same year, CrossRef applied DOIs to journal articles. However, it took another 10 years for non-article output to receive DOIs in aggregators like DataCite. DataCite allowed for DOIs to be registered for data and other output, like computer software.
Furthermore, a movement advocating research datasets grew, aiming to increase awareness and improve practices regarding the publication of datasets. This is best represented by the FAIR (findability, accessibility, interoperability, and reuse) and Joint Data Citation principles.
This movement was necessary because, increasingly, funding agencies like the Wellcome Trust and the Gates Foundation started including data sharing as part of their funding policies. Also, increased research and development spending across the world is accelerating the generation of data.
What this means for Open Access science
The Open Access movement is typically associated with the publication of scholarly articles without any restrictions such as subscription fees. The growth of non-article output, and requirements of certain agencies to openly publish data, could lead to the development of a new open science ecosystem.
This would involve not just the free availability and usability of scholarly articles but also the data and methodologies, including code or algorithms, that were used to generate those data.
However, for data to be valuable, it needs peer reviewing. Given that peer reviewers are already burdened by their workloads, why should they include data peer review as well?
The value of data peer review
Here are some of the benefits of publishing peer-reviewed data.
- Transparency: By publishing the data alongside the results, peers can understand the thought process of researchers and see whether the data used are sufficient for the conclusions drawn.
- Cooperation: Scholars from differing fields, who may not be able to review the data themselves, can rely on peer-reviewed data when applying it to their respective disciplines.
- Potential for automation: Reviewed data can be uploaded to databases that feature artificial intelligence-based software that can detect patterns and provide search features.
Basically, peer-reviewed data would benefit various disciplines and help boost interaction between them. It would make it easier for researchers to replicate and reproduce reported work and strengthen scientific rigour and reliability.
For example, fields in biomedicine and psychology could share data across different specialities to expand their studies. Moreover, citizens could contribute data to fields like astronomy and ecology by submitting photos or information that AI could analyse.
What data peer review looks like
Presently, data peer review remains undefined. It mostly looks like normal peer review but has more complicated features. We must look at common patterns in the journals that do practice it.
Generally, there’s a focus on the appropriateness and quality of the data, the metadata quality, and the suitability of the data. Other factors include opportunities for reuse, links to public repositories, and descriptions of how to use it.
Let’s look at a specific example.
Data journal’s guidelines
The MDPI journal Data publishes Data Descriptors. These are articles that publish descriptions of scientific and scholarly datasets. Data scientists and scientists working with data are the intended audience of these pieces.
The data peer review centres around three key points, which are supported by questions:
- Data description: originality, well-defined source, reproducibility, metadata and disciplinary standards, copyright license.
- Data quality: dataset, appropriate control measures, possible sources of error and noise.
- Data access, archiving, and metadata: DOI number, format, reusability.
Similar guidelines are seen in other MDPI journals too, like Software and Analytics. And they are supported by TOP Guidelines and FAIR Principles.
But what’s stopping all journals from implementing data peer review?
Challenges of data peer review
For data review to spread, it would be a challenge to an already strained peer reviewing system.
Peer reviewing data is a complicated process that requires different approaches. It requires different expertise than traditional articles, including knowledge of data structures and metadata standards. However, it also requires specialist knowledge from the field the data is from to verify its quality and relevance.
Accordingly, data peer reviewers should have a wider disciplinary distribution than the pool of typical scientific articles. This requires innovation. Database hosts, journals, academics, and funders need to work together to create a sustainable model for peer reviewing data.
As we’ve already addressed, the benefits of data peer review are great and, with the rate data are being generated at, it is becoming an increasingly necessary part of the peer review process.
Integrating data peer review
By introducing the peer review process to data and other non-article research output, we can increase the potential for transparency, cooperation, and automation in scientific publishing. Additionally, it could help broaden the Open Access movement into becoming a more encompassing ecosystem of research that would fuel the capabilities of AI.
How can we begin integrating the peer review of data and other non-article research output?
The first step is recognising the hard work scientists are already doing in creating and reviewing non-article research. This is why Peer Review Week is so valuable: it lets us celebrate the historical process of peer review and all the scientists who are dedicated to upholding it.
If you want to explore Peer Review Week more, we have plenty of content that will interest you. See our article Peer Review Week 2023 here.