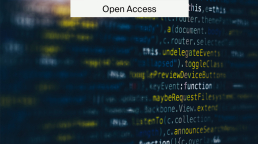
AI is Changing Open Access Science
ChatGPT took the world by storm, and its technology has only improved since. The potential that AI offers is shaking up entire industries, but how is it changing Open Access science?
Here, we’ll look at some of the challenges that Open Access scientific publishing is facing, how AI can help, and what we need to watch out for as it’s being increasingly implemented.
Acceleration
In another MDPI Blog article, we discussed how the idea of “acceleration”, which was key to early discussions of the Internet, was used by early Open Access supporters.
The Internet was described as being capable of rapidly producing change and results, whilst academia was presented as rooted in centuries-old tradition. From this, Open Access would remain in touch with traditional knowledge production but using revolutionary technology.
Open Access embraces the new without severing from the old, and it also accelerates the old using the new.
Open Access can lead the way
Artificial intelligence, like any new technology, presents both a threat and an opportunity. It requires reflection, adjustment, and adaptation.
If implemented carefully and thoughtfully, AI could help us respond to some of the issues that the open access scientific publishing industry currently faces, including the increasing amounts of data being produced and language barriers and imbalances in outputs between countries, and help to promote openness in datasets and content aggregators. It can also be implemented to help boost the potential of interdisciplinary research by bridging skill gaps and automating tasks.
Coping with the volume of data
Currently, the number of scholarly articles being published, and the amount of data that goes along with it, surpasses what anyone can read or effectively manage. This affects readers, writers, and publishers alike. The work just gets lost in all the noise.
That’s why search engines, content aggregators, and databases are so valuable. They help people find what they need. How can AI assist in this?
Implementing AI in scientific publishing
To implement AI, you should begin by identifying problem areas. This can include tasks that are time-consuming, ones with lots of data, and repetitive administrative tasks. After identifying them, we should look for ways to measure these tasks using data, because data is fuel for AI, improving its accuracy and ability.
Here are some examples of how AI could automate certain data-related aspects of the publishing process:
- Keyword searching for suggesting relevant journals and search engine optimization.
- Analysis of datasets to generate insights and identify new research areas.
- Processing data for content aggregation in databases.
- Providing advanced search options in databases.
- Checking for and removing duplicate articles in databases.
- Correctly labeling and organizing articles.
How well the AI you are implementing performs depends on the quality of the data you train it with. Implementing AI requires preparation and organization, but if done so effectively, it will produce results quicker and quicker.
Language barriers in publishing
Open Access can help us to establish a global scientific knowledge base, but there are many barriers for non-native English speakers.
Around three-quarters of scientific papers are published in English, but English speakers only make up 5% of the global population. This means that most academics read and write papers in a second language, making translating tools highly valuable to speed up this process.
AI as a translator
Nowadays, many translators incorporate elements of AI. Google Translate has AI-powered features that enable it to learn and adapt more as it’s used. AI models like ChatGPT can make the experience of translating interactive by using Natural Language Processing (NLP).
NLP applies computational techniques to analyze human language and produce human-like responses. ‘GPT’ stands for ‘Generative Pre-trained Transformer’. ‘Pre-trained’ refers to how the model is trained on a large corpus of text data to predict the next word in a passage.
Having been trained on so much natural text means that GPTs can provide contextual awareness to translations. Moreover, their conversational format means researchers can ask for clarity, synonyms, or alternative translations to words and phrases. Thus, this would make the translation more like a conversation.
GPT is not fully reliable
GPT training involves analyzing a huge body of text and noticing patterns so it can predict the next word in a passage. This results in human-like text, meaning it sounds like it’s written by a human but may not necessarily be by one.
AI is changing Open Access by helping to lower the barriers to non-native English speakers. It must be used intentionally and specifically in response to problems, keeping in mind how it works and what issues this may cause down the line.
Highlighting the case for openness
One of the biggest obstacles to Open Access is a lack of awareness or misunderstandings about its aims. Artificial intelligence could help with this. AI needs to access data to work. It often interacts with Open Access scientific data, and AI is frequently being developed with Open Access principles in mind.
During the COVID-19 pandemic, AI and Open Access converged in the COVID-19 Open Research Dataset (CORD-19). This highlighted the potential of Open Access in dealing with crises.
CORD-19
Backed by the US White house and several institutions, CORD-19 applied recent advances in NLP and machine learning to biomedical research to provide almost complete coverage on COVID-19, coronaviruses, public health, and more.
It applied text mining and information retrieval to a rich collection of data and structured full-text papers, mobilizing scientists by providing them with quick and up-to-date access to research.
CORD-19 was designed for the biomedical community. However, it demonstrates the general potential of bridging computing and scientific communities around a common cause. Its success shows that if scientific literature were widely available for AI analysis, it could potentially speed up advancements and help to fight crises like the COVID-19 pandemic.
AI is changing Open Access
AI is changing Open Access; it’s changing everything. Ultimately, though, it’s a tool, so how it’s used determines its value.
If used carefully, AI could help Open Access by automating repetitive data-related tasks, making the translation process more interactive, and promoting openness in datasets and content aggregators. However, attention must be paid to its flaws and potential for misuse.
Evidently, it’s an exciting time for Open Access as we enter this technological revolution. If you want to learn more about Why Open Access is Important, we have plenty of articles that may interest you.