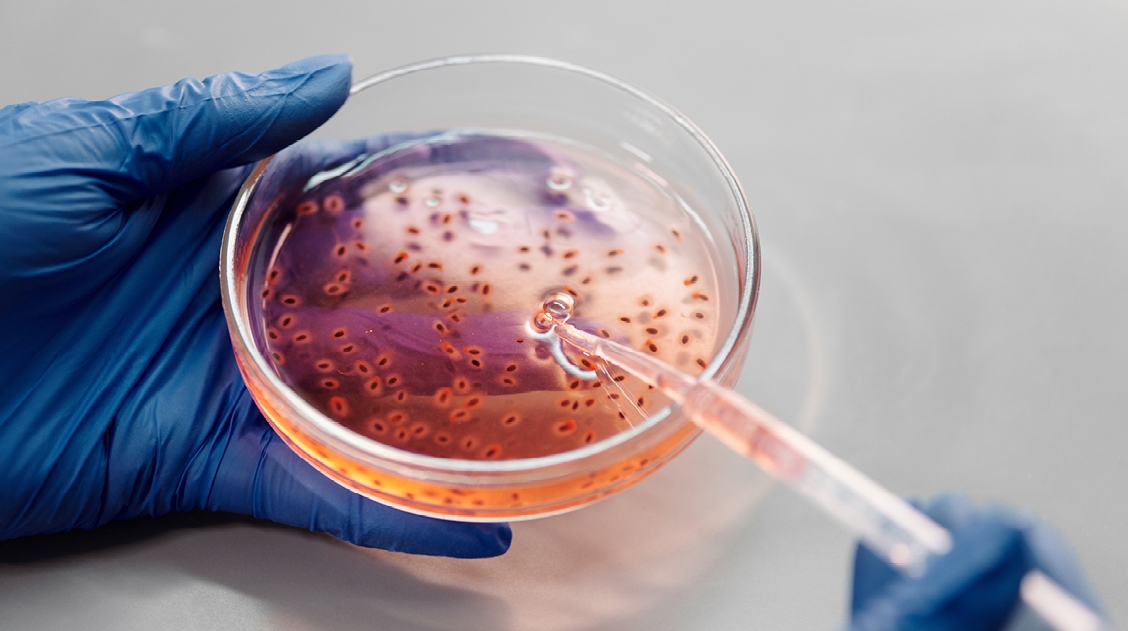
Predicting Glycosylation for Therapeutic Antibodies
Glycosylation, the post-translational addition and processing of covalently bound oligosaccharide chains, forms glycoproteins. Variations in the glycoform of proteins result from multiple factors in this process, such as enzyme and substrate availability. These differences can influence the stability and biological function of the protein.
In recent years, antibodies are increasingly utilized to treat disease, and there is thus a broad interest to optimise the engineering of these glycoproteins for increased therapeutic value. In their article, Jedrzejewski et al., present a modeling framework that links the availability of specific nucleotide sugar substrates in extracellular growth media to intracellular metabolism and antibody glycosylation.
Author Cleo Kontoravdi discusses this work below:
The advantages of an silico model of antibody glycosylation
Cleo Kontoravdi: It is now possible to create large sets of bioprocessing data in both industrial and academic settings. Mathematical models can help analyse such datasets across scales, integrating different ‘omics technologies, in an effort to identify sources of variation and perform in silico experiments.
The model of antibody glycosylation presented in this work has three main components: a cell culture model, a structured metabolic model and a description of the glycosylation process in the Golgi apparatus. By linking the three we are able to evaluate (a) the effect of changes in the cell culture environment and (b) the effect of nucleotide sugar transporters and glycosyltransferases expression on protein glycosylation.
The model can therefore aid both process development in terms of formulating appropriate feeding strategies, as well as guiding genetic engineering strategies to improve and control the glycoform.
Building the glycosylation model and platform development
CK: We first started by building the antibody glycosylation model and comparing it to a number of experimental data for various commercial antibodies. The next challenge was to link this to a metabolic model for nucleotide sugar synthesis. We first attempted this at steady-state and then went on to include an overarching dynamic cell culture model. The final product is able to give us dynamic results over the cell culture period. In-house dynamic experimental data were essential to the platform development process.
Findings and challenges and next steps
CK: The main contribution is the modelling framework, which can be transferred to other cell lines and products with a small number of experiments. The biggest challenge is to prove that this framework can be used to direct process design and genetic engineering approaches not only for antibodies but also for more complex glycoproteins. The next steps are to mode to a CHO cell experimental platform and to build on the contribution of Krambeck and Betenbaugh for considering more complex glycan structures. We are also working on confirming the fidelity of in silico results under a wider range of conditions.
About Cleo Kontoravdi
Cleo Kontoravdi holds M.Eng. and Ph.D. degrees in Chemical Engineering from Imperial College London. After working as a Research and Development Scientist at Lonza Biologics, she returned to the Department of Chemical Engineering at Imperial as a Lonza/RCUK Academic Fellow in Biopharmaceuticals Processing, where she is currently a Lecturer.
Her interests lie in the area of biotechnology, with particular focus on the application of systems engineering principles to bioprocessing. Her research involves the systematic integration of model-based tools, such as sensitivity analysis. It also includes design of experiments and optimization, with experimentation on mammalian cell culture systems. Topics of interest include optimization of culture media and conditions, protein glycosylation, amino acid transport, metabolic flux analysis and multiscale modeling.
Further reading
To learn more about the content of this article, we refer you to the following:
- Jedrzejewski, P.M.; del Val, I.J.; Constantinou, A.; Dell, A.; Haslam, S.M.; Polizzi, K.M.; Kontoravdi, C. Towards Controlling the Glycoform: A Model Framework Linking Extracellular Metabolites to Antibody Glycosylation. Int. J. Mol. Sci. 2014, 15, 4492-4522.
- Krambeck FJ, Betenbaugh MJ. A Mathematical Model of N-linked Glycosylation. Biotechnol Bioeng.2005 Dec 20;92(6):711-28.