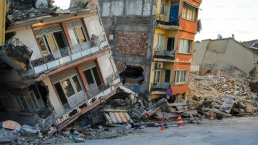
AI Model Developed to Predict High-Risk Areas of Soil Liquefaction Caused by Earthquakes
Novel research from the Shibarua Institute of Technology in Tokyo centred around developing a model that predicts the risk of soil liquefaction in areas within Yokohama, Japan. This model used artificial intelligence (AI) and machine learning (ML) technologies. Ultimately, this model was designed to potentially reduce the effects of earthquakes and other natural disasters.
An author of the study, Prof. Inazumi, emphasises this point:
We were motivated to pursue the research after we recognised the urgent need to improve urban resilience to earthquakes, especially in rapidly urbanizing areas prone to seismic activity – Prof Inazumi, an author of the study
The study is published in the Open Access journal Smart Cities and may play an important role in the future planning and development of infrastructure in urban areas. The researchers aimed to generate a soil liquefaction risk map of areas within Yokahama based on geographical and geotechnical data.
What is soil liquefaction?
Soil liquefaction occurs when a significant amount of force is applied to saturated soil, resulting in instability and a loss of strength. This force usually comes from natural disasters such as earthquakes.
Specifically, liquefaction occurs when a greater amount of water is present, and the soil particles are spread apart due to shaking from earthquakes. This results in them becoming substantially less dense and more loosely packed.
Hence, this results in significant weakening that causes the ground to no longer support infrastructure such as buildings and houses. Japan is a country that is highly susceptible to experiencing earthquakes, with the city of Yokohama being particularly prone to the potentially devastating natural disaster.
Measures are constantly being developed to mitigate the destruction caused by earthquakes. This includes ensuring that construction is carried out on soil that does not result in liquefaction and, hence, the destruction of buildings.
Currently, traditional methods solely rely on human judgment to evaluate soil quality and the risk of liquefaction. Therefore, using AI and ML technologies is instrumental in developing more advanced and sophisticated measures for soil liquefaction mapping.
Using machine learning and neural networks
The research in Smart Cities used different types of ML techniques to create a model for the local area. This includes the use of artificial neural networks, a deep learning process that involves the mathematical models that computers use to solve complex problems. The model is connected by nodes, or neurons, and processes information similar to how the human brain would. This type of model can examine data from geological surveys and seismic records (records of the ground vibrations and movements caused by earthquakes).
Furthermore, the researchers also used gradient-boosting decision trees. These are highly accurate and efficient techniques to make predictions from data. As decision trees are often prone to making errors, multiple decision trees are combined, hence called ‘boosting’, providing much more accurate and reliable results by reducing the errors made.
Since traditional methods for predicting soil liquefaction are often limited by the scale of data integration and speed of analysis, resulting in gaps in emergency preparedness and risk management, we decided to leverage advanced technology such as AI and ML to develop a more dynamic and accurate predictive model – Prof. Inazumi
AI model to predict soil liquefaction
To generate a risk map within the Yokohama area, the researchers designed the model to predict the following ‘soil type classifications’:
- Sand and gravel (with fine content 35% or less)
- Silt
- Clay
- Bedrock (with fine content of 50% or more)
In addition, the model categorised the soil based on important N-values which are critical in determining the extent to which the soil can bear loads. For example, an N-value of 20 or more is suitable to bear a load. However, a value of 50 or more is highly solid and suitable to support large buildings and heavy infrastructure.
The study also included the groundwater level as a soil property, which impacts the soil liquefaction risk. Furthermore, it took into account different earthquake motion characteristics. Based on the predicted data, the model then categorised the liquefaction potential index (LPI) to evaluate the liquefaction risk in different areas in the Yokohama region.
To test the accuracy of the prediction models designed to determine N-values and soil classification, the authors ran training tests from different sites and ground depths. Here, they found that data obtained from ‘20 meters below the ground to 1 meter above ground’ were the most accurate. Furthermore, they found that the more available data for the model during training tests, the more accurate the prediction would be.
Importantly, the soil liquefaction maps generated from this AI model proved highly accurate and efficient compared to current methods of determining liquefaction risks.
The real-world application of our research is the development of hazard maps which can help urban planners and engineers to visualize and identify areas at high risk for soil liquefaction and make informed decisions regarding the development of infrastructures. – Prof. Inazumi
The innovative study emphasises the importance of predicting soil liquefaction during earthquakes. This is highly important, particularly for earthquake-prone countries when planning infrastructure for urban areas and promoting urban resilience.
To read more about novel science and technology developed for urban areas, explore MDPI’s Open Access journal Smart Cities, or click here for a full list of journals.