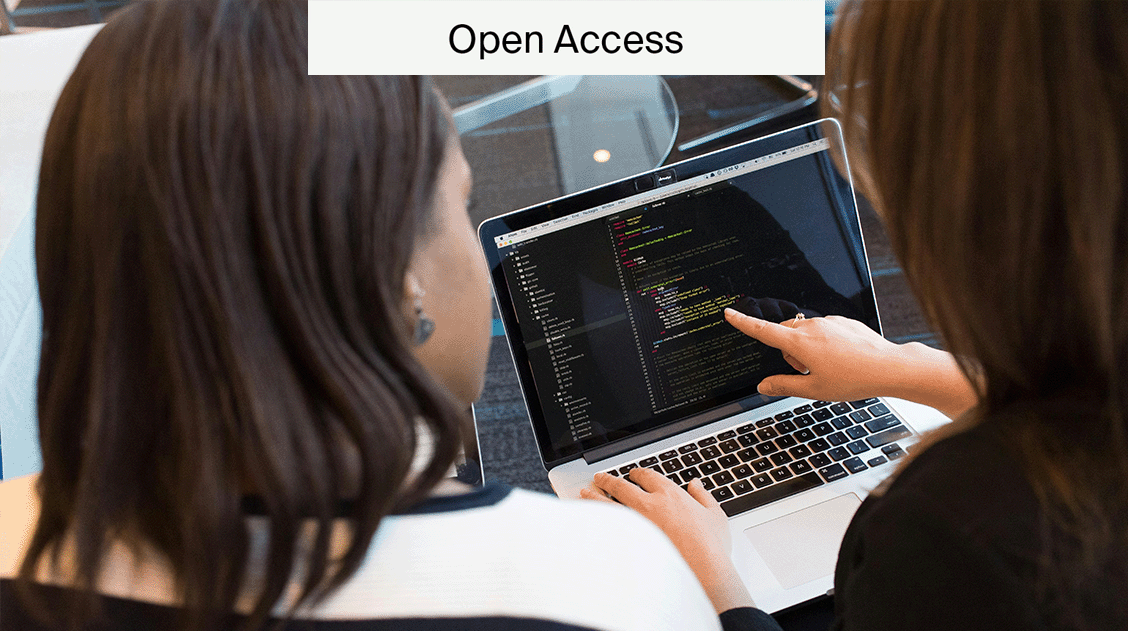
Open Science Principles Can Improve Artificial Intelligence
Artificial intelligence (AI) tools are already being used by researchers to tackle challenges related to healthcare and climate change. However, there are concerns across the scientific community that AI is damaging reproducibility and trust. As open science principles are increasingly being embraced, there is an ongoing conversation about how AI can support these principles whilst addressing the challenges of trust and reproducibility it poses.
We attended the UNESCO event ‘Navigating the Intersection of Open Science and AI’. The event focused on ‘exploring opportunities and challenges in the era of artificial intelligence’.
Here, we discuss how open science principles can be applied to artificial intelligence, so that each supports the other. We’ll also expand on insights from the discussions at the event.
UNESCO and open science
The United Nations Educational, Scientific and Cultural Organisation (UNESCO) is an agency of the United Nations (UN) that aims to promote international cooperation in education, arts, sciences, and culture. UNESCO advocates for open science and has published a widely influential set of recommendations to further establish it.
Here is a summary of the UNESCO open science recommendations:
- Promote a common understanding of open science, its pros, cons, and diverse pathways.
- Develop an enabling policy environment.
- Invest in open science infrastructures and activities.
- Invest in human resources, training, education, etc.
- Foster a culture of open science and align incentives.
- Promote innovative approaches along the scientific process.
- Promote international and multi-stakeholder collaboration.
But how does AI relate to this?
How researchers use AI tools
A recent study performed by Oxford University Press found that most researchers and research authors say they are using AI tools in their research practice.
The study surveyed over 2000 researchers across geographies, subject disciplines, and career stages. Of them, 76% use AI tools, particularly machine translation and chatbots. Also, 67% feel that these tools benefit them in some way.
AI is very useful when it comes to improving writing and translation. However, employing AI technology can lead to misapplication in other parts of the scientific process. For example, generating summaries of articles could introduce errors into the introduction or abstract of the article.
In 2023, the annual number of papers retracted by research journals topped 10,000 for the first time. Alongside the growth of fake papers and paper mills, AI tools have raised concerns across the scholarly community for the potential of their misapplication, intentionally or not.
Applying AI to data
Professor Alison Noble, University of Oxford, provided the keynote address for the event. She is an interdisciplinary researcher developing machine learning solutions to key problems in biomedical analysis.
She describes how AI is very useful for analysing large datasets, modelling data, and combining different data sets. All of these uses can lead to the discovery of new patterns and insights. AI is particularly useful in fields with abundant data, like healthcare.
However, she explains, scientists struggle to obtain reliable access to high-quality data. Ultimately, the accuracy of AI prediction depends on having high-quality data, leading to the ‘garbage in, garbage out’ concern—if you input poor-quality data into AI, its outputs will be poor quality too.
Incomplete, incorrect, or unrepresentative data can have a big and harmful impact, as AI can produce outputs reflecting the data’s errors. In medical diagnoses, for example, this is dangerous.
Determining the accuracy of AI prediction depends on researchers’ abilities to judge the quality of the datasets that they input into them. Furthering this is that most AI tools are black-box systems, meaning that their internal workings are not understood by users and sometimes even by developers.
Why can’t researchers understand how AI tools work?
AI tools, like Large Language Models, are developed by training them on huge amounts of data and content. This means that they can repeat negative patterns found in their training data, such as biases, lack of attribution, and gaps in coverage.
The largest and most used AI tools are developed by private companies, who have no incentives to release information on how their tools are trained and what data are used. This lack of clarity makes it very difficult to understand the tool’s inner workings and subsequently what its strengths and weaknesses are.
In science, this ultimately damages the reproducibility of findings from black-box AI tools, and can lead to the use of unattributed work and reduce the overall transparency. All these effects negatively impact the openness of science.
Applying open science principles to artificial intelligence
In the event’s central discussion, the issue of AI tools negatively impacting open science was discussed. The solution, highlighted unanimously by speakers, depended on interdisciplinarity and developing incentives.
Interdisciplinary research
To improve AI tools in line with open science principles, Professor Noble explained how we need to foster innovation at the intersection of researchers and AI developers. This requires experts from both sides working together.
Laura Joy Boulos, L’Oreal‒UNESCO International Rising Talent, outlines how researchers are more capable than AI developers for judging, testing, documenting, and managing tools for use in science. Accordingly, she suggests, the research community must be a partner in the development of fit-for-purpose AI models.
Further, if such collaboration is supported by the open sharing of results, the community can continue to build on vital work at the intersection between AI and science. There would be a common conversation that could bring diverse perspectives together around how to better improve AI tools.
Open science enables the interdisciplinary work that is necessary for improving AI by ensuring that research is accessible and there are limited barriers when building on it.
Other obstacles to interdisciplinarity
Other obstacles include the isolated environments that AI tools are developed in and also the lack of incentives for researchers to become partners in development.
Firstly, the discussions suggested there needs to be policies and frameworks produced by companies and governments to incentivise private companies to let researchers scrutinise these tools. Second, as Professor Noble explained, there needs to be a system rewarding researchers for working on AI models.
Creating incentives for AI research
Testing AI tools is a time-consuming process. It involves validating and labelling data, testing models and their outcomes, and many other tasks to ensure accuracy. Furthermore, learning how to do these things requires training in and knowledge of AI and computer science, alongside the knowledge relevant to the fields the data are from.
Researchers are not rewarded for such work; there are no incentives for testing AI tools. Instead, when seeking jobs or promotions, academics tend to be evaluated on their publication history. This has led to the so-called ‘publish or perish culture’.
Creating incentives for things like sharing data and negative results, developing and testing AI tools, and providing feedback for and information on use cases of popular models would encourage scholars. And, if shared openly, researchers can exhibit the vital work they are doing by helping develop AI tools.
The central recommendation by Professor Noble is to incentivise more co-designing of AI tools, rather than having researchers be passive users. This will hopefully increase their accuracy, transparency, and improve knowledge translation.
Key to this is sharing the results of the co-design process openly, as Professor Noble explains
[We should build a] community of practice around reproducibility … learn together how to do this in the best possible way, developing this culture alongside a strong scientific culture.
Arguably, you could summarise the event’s arguments by applying the UNESCO open science recommendations outlined above as the steps to improve AI:
- Promote a common understanding of artificial intelligence, its pros, cons, and diverse pathways.
- Develop an enabling policy environment.
- Invest in AI infrastructures and activities.
- Invest in human resources, training, education, etc.
- Foster a culture of open AI and align incentives.
- Promote innovative approaches along the scientific process.
- Promote international and multi-stakeholder collaboration.
How open science principles can improve AI
Applying open science principles to the development of artificial intelligence tools is vital. This is because it would help bring together diverse perspectives from scientific disciplines and AI developers so they can actively address the concerns and limitations of the currently used AI models.
Interdisciplinarity is necessary for improving AI tools and ensuring they align with scientific principles. However, we need openness to enable such collaboration and a system of incentives to encourage researchers to perform the work. As such, the speakers advocate for a top-down approach, setting up incentives and requirements that will help researchers be involved in codesigning AI.
The UNESCO event outlined the vital importance of establishing open science principles as we experience the changes caused by AI’s growth. Overall, the speakers highlight the value of fostering an environment that values transparency, collaboration, and the open sharing of knowledge. The value being it could help us tackle the world’s most pressing issues.
Also, we’ve covered MDPI’s policy and approach to AI in various articles. For example, we’ve covered the AI tools we offer researchers and also publication ethics.
If you want to learn more about Open Access, see our article Why Open Access is Important for more.